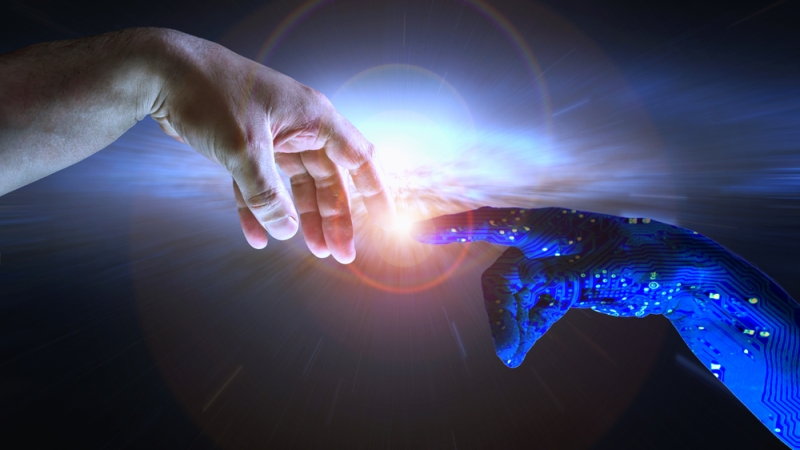
Machine learning and artificial intelligence are needed to bridge the gap between the volume of government intelligence data and the number of people capable of analyzing it, according to Jason Matheny, director of the Intelligence Advanced Research Project Activity (IARPA).
“The world has scaled up in complexity, though, at a rate that outpaces the number of human brains to deal with that level of complexity. We’re well past the point where it’d be possible for thousands of intelligence analysts, or 17 agencies with thousands of intelligence analysts, to make sense of all of the complexity. So we do need to find ways of leveraging tools in order to break down that complexity into pieces that the humans can better understand,” said Matheny at the IBM Government Analytics Forum on June 1. “There’s a good reason to think that machine learning in various applications can help to bridge that gap.”
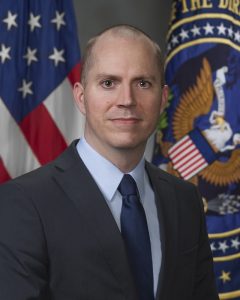
According to Matheny, intelligence data should be categorized into that suited for machine analytics and that suited for human analytics. That way, teams of computers and people can parse through the data efficiently and effectively.
For example, Matheny described analyzing a picture of military tanks as a case for a machine-human team. Artificial intelligence can determine how many tanks there are, and potentially where they’re located, while a human analyst can make determinations about why those tanks are there and what it means for the government.
“There’s a lot that can be done to leverage existing tools and automate some aspects of national intelligence, and then an analyst is spending less time on tasks like finding or counting tanks, and more time thinking about why the tank is there at all,” said Matheny.
However, Matheny said that though nearly half of the projects funded by IARPA have something to do with machine learning, there are fundamental problems with current capabilities that have to be resolved.
First, Matheny said that current supercomputers use approximately a million times the energy as the human brain to complete similar tasks with lower levels of performance.
“How is it that the brain is able to compute so efficiently and so well on sparse learning tasks?” said Matheny, adding that IARPA is working on a program to reverse engineer how the brain computes.
Matheny said that artificial intelligence also needs to improve its determination of cause and effect, particularly when it comes to analyzing rare or extreme events. And the system needs to be able to explain how it came to a conclusion to a human operator.
“An analyst will not use the system unless it understands how a result is generated and it can defend that result to a policymaker,” said Matheny.
Finally, there is concern over how an AI system may be spoofed, through altering select pixels in an image or other small data points. These spoofs can have serious consequences if the intelligence gathered by such systems is used to inform government and military policy.
“The government will not solve these problems alone,” said Matheny. “Industry investment, which is also increasing much faster than government investment is going to be critical here, and we need to find ways within government to best leverage those investments that industry is making.”