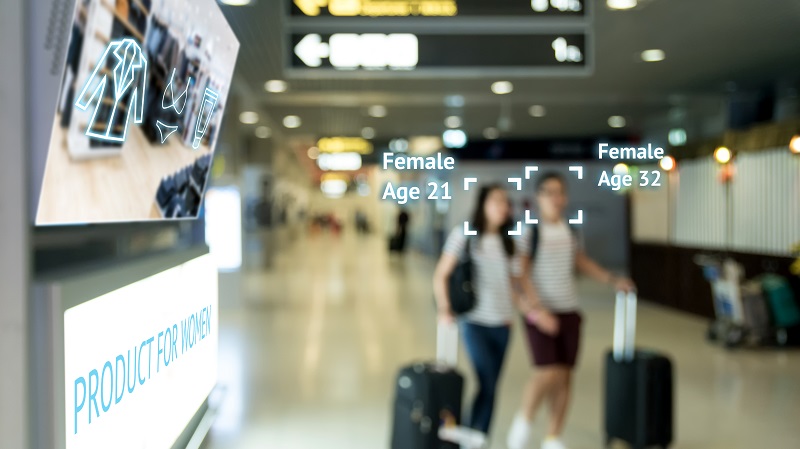
In a recent report on facial recognition algorithms, the National Institute of Standards and Technology offers Federal agencies a map of the time, costs, and manpower associated with processing thousands of faces.
The National Institute of Standards and Technology released its Face In Video Evaluation (FIVE) program report on March 7. The FIVE program, established in 2014, tests how well different facial recognition algorithms identify people appearing in video sequences.
For this report, FIVE tested 36 algorithms supplied by 16 commercial companies that specialize in facial recognition technologies. These companies sent NIST their algorithms in December 2015; Patrick Grother, one of the NIST scientists behind the report, said he and his team finished running the algorithms about 12 months later.
“The report argues that this is complicated because there a number of different factors,” Grother said in an exclusive interview with MeriTalk. “If it’s to be a success, you need a multi-disciplinary team. You might change the environment to try to arrange for subjects to look at the screen. It’s not one size fits all.”
To conduct the study, Grother and fellow NIST scientists George Quinn and Mei Nan tested the algorithms against reams of archived footage from seven datasets to see which were most accurate. Videos from fixed cameras were scanned against portrait-style photographs of up to 48,000 enrolled identities. Different locations were represented through the datasets, including the passenger gate at an airport, a baggage pickup area, and a sports arena.
The study used volunteers walking in three different patterns. The cameras captured unidirectional flow (crowd moving one way), bi-directional flow (crowd moving two ways), and winding queue (people lined up at a refreshment stand).
Grother explained that facial recognition research is divided based on three types of subjects: cooperative, non-cooperative, and uncooperative. Cooperative subjects are those who are knowingly looking at a camera straight-on, as they would for a passport photo. Non-cooperative facial recognition means a person can be facing off-angle, with his or her face obscured. Identifying uncooperative subjects, people who obscure their faces with sunglasses and ball caps, is hardest of all, Grother said.
Face detection technology generally works best on cooperative subjects. However, FIVE assessed non-cooperative facial recognition. Non-cooperation requires unknown video imagery to be compared with images previously collected from multiple individuals.
“Accuracy depends on the number of people enrolled in a database,” Grother said. “The problem is quite hard if you don’t have any cooperation from subjects.”
The report, which was supported by the Department of Homeland Security’s Science and Technology Directorate, states that “there is a massive variation in accuracy between algorithms”. It says that non-cooperating facial recognition can only be as accurate as still photograph recognition when it is captured with the same high quality as photographs.
“Further, high accuracy can only be achieved by deliberate installation and con-figuration of cameras and the environment, and such control over the deployment may sometimes be impossible, for physical, economic, or societal reasons,” the report states.
Most videos display 24 to 30 frames a second, and Grother said processing these images is a long and costly process. The more people in the video, the longer it takes to process a video.
For example, Table 33 in the FIVE report reveals that processing a video image with no people and no motion takes 23.89 seconds when using algorithm A30V. Using the same algorithm, processing a video image with between one and three subjects takes 39.95 seconds. The algorithm, when applied to a video image with four to seven subjects, processes the image in 61.97 seconds.
The report also states that, while multiple cameras provide more benefits, the cost of computation increases linearly with the number of cameras. According to the report, it is almost certainly less expensive to “deploy a capable attractor with eye catching and varied content” than to use multiple cameras.
“That’s an expensive process for some algorithms,” Grother said. “The importance of that is in the amount of hardware they need to buy. It also depends on the price of the searches you need to conduct.”