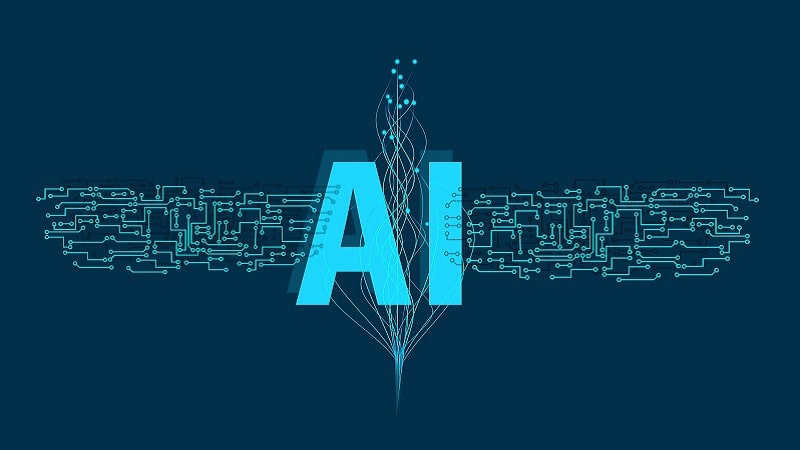
As Federal agencies across the government are looking to scale up the use of artificial intelligence, former Federal officials are emphasizing the importance of data and having the right processes and governance in place to help facilitate that scaling.
In a March 23 panel at NVIDIA’s GTC Conference moderated by former Federal CIO Suzette Kent, former officials charged with leading the AI change at the Department of Defense’s (DoD) Joint AI Center (JAIC), the Central Intelligence Agency (CIA), and the United States Postal Service (USPS) shared the lessons they learned scaling AI practices at their respective agencies.
In addition to keys such as needing a champion in leadership to help “hack the bureaucracy” – as former and first-ever JAIC Director retired Lt. Gen. Jack Shanahan put it – officials repeatedly came back to the importance of having the right data, data governance structures, and processes in place to properly move projects from the pilot phase through implementation.
Shanahan was one of the team members charged with starting DoD’s Project MAVEN, which brought AI and machine learning from the theoretical to the battlefield. His work on the project was one of the reasons he was chosen to be the JAIC’s first director, where he served until his retirement in 2020.
“I go back to the very early days of our MAVEN journey. We didn’t know what the stack was. We didn’t know enough about it to say here’s what the AI stack should look like,” Shanahan said. “But I’ll tell you after every step of the journey, after two years, we’d learned what you needed to bring AI pipeline together all the way from that initial data collection … all the way to fielding a model, and then sustaining that model, which a lot of people tend to forget.”
In order to do that, Shanahan said the JAIC had to build a data management pipeline, a platform, and a data protection strategy in order to have the processes in place to properly scale AI across the JAIC.
“We knew what the platform needed to look like, what the infrastructure-as-a-service needs to look like, what the platform-as-a-service needed to look like, what the data, what the tools, what the libraries all needed to look like,” he said. “We could start building that to bring it to scale in the JAIC, and it was a journey of discovery.”
Shanahan also pointed to the need for “just getting started” sometimes, which Kent backed up. Kent added, “Getting started is how you learn, and you get the capabilities, people, and technology to move forward.”
Former CIA Deputy Director for Science and Technology Dawn Meyerriecks also emphasized the need for proper data, as well as the need for stakeholders to have trust in the processes to be able to properly scale AI.
“We have a long history of having very stovepipe systems. They’re exquisite, but we don’t generally start a problem thinking about how we’re going to share data from those particular collectors,” Meyerriecks said.
Once they were able to get the proper data and begin feeding them to AI and machine learning algorithms to operate at scale, it was then important that the people using that data were able to trust it.
“We had to really establish with our analysts that they could trust what was coming out of our analytic models,” Meyerriecks added. “And I can’t underscore the value of that and how important that was early on. So, it wasn’t just the provenance of the data, but it was also showing them the homework.”
Kristin Seaver, who developed the AI/ML roadmap at USPS as the CIO and executive vice president from 2016-2020, said that it was key that she and leadership understood that to properly implement AI at scale and make AI solutions usable for the disparate teams that needed access to them across the agency, the processes and governance needed to be overhauled to properly fit the new use cases.
“One of the things we had to really revisit was some of our policies around data and who would have access, and how data could be used,” Seaver said. “Those had to be relooked at and in some cases updated, because when they were written, frankly, the possibility of AI in our workspace just wasn’t even palatable for people. You couldn’t even think about the use case. So that was critically important, and you’re gonna have to continue to do that.”
“AI is a is an iterative process,” Seaver added. “So, understanding that it’s going to take cycles of refinement and learning to get closer to the solution you’re looking for.”