Look Who’s MeriTalking: Fighting Fraud, Waste, and Abuse With Big Data
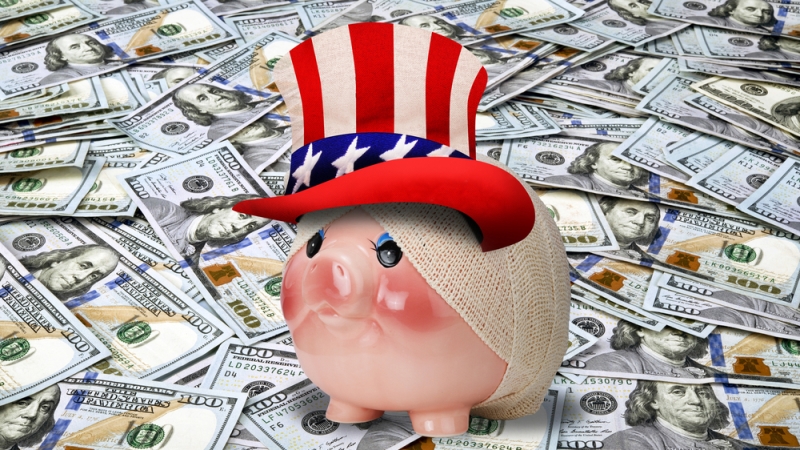
(Image: Shutterstock)
MeriTalk recently spoke with Alan Ford, director of Teradata Government Systems. He delved into fraud, waste, and abuse in the government–why it happens and how big data and machine learning can play a role in stopping this $300 billion-a-year problem.
MeriTalk: Why do we see fraud, waste, and abuse continue to rise–especially related to Medicare and Medicaid–when reducing them has been a sustained priority?
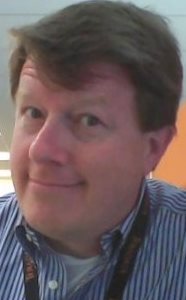
Alan Ford: Medicare and Medicaid, specifically, are classified as high-risk programs by the Federal government as they have a greater vulnerability to fraud, waste, and abuse mismanagement. There are a couple of reasons for this. First, traditionally, the penalties in this area have been low relative to other crimes and they are nonviolent crimes, so deterrence is low. Second, the system operates in a pay-first, check-later fashion, making it more susceptible to abuse because barriers to entry are low and the perpetrators are often long gone once the fraud is discovered. Third, Medicare and Medicaid are vital programs, so any changes to make fraud detection easier have to be done without interrupting the delivery of lawful recipients’ services.
Medicare and Medicaid comprise just one area of fraud, waste, and abuse. There are many more similar use cases including Federal student loans, defense contractors, disaster relief requests, and mortgages. Many of the same issues that put Medicare and Medicaid at risk apply to these other areas as well.
MeriTalk: Where has the greatest progress been made in stopping or reducing government fraud, waste, and abuse? Are there specific programs that you can cite as best practices?
AF: The more data that is made available for analysis, the better the chances that agencies can generate adequate levels of information to drive the detection of fraud, waste, and abuse. Data sharing across Federal and state barriers enables new insight into fraudulent activity, which is difficult to achieve when data are kept siloed. Data sharing is a major opportunity for agencies to become more effective.
As an example, the Health Care Fraud Prevention and Enforcement Action Team (HEAT), a joint task force among Health and Human Services (HHS), the Department of Justice (DoJ), and the Office of Inspector General (OIG), was created to share data and information. Since its inception in 2009, it has detected and collected more than $7 billion of fraudulent monies and convicted almost 2,000 different defendants–very effective work.
Another best practice program has originated from the Centers for Medicare & Medicaid Services’ (CMS) Integrated Data Repository (IDR), one of the largest and most successful fraud and health care information repositories in the Federal government. It is based on a high-volume data warehouse, including information such as Medicare beneficiary data, provider data, contract information, and risk scores. The combination of these data sources into one integrated environment empowers organizations such as HEAT to use the data to generate new insight. Teradata has helped CMS run and operate IDR for more than 10 years with extraordinary results.
MeriTalk: What role can and must big data and analytics play in reducing the incidence of fraud, waste, and abuse–including preventing improper payments and determining accurate eligibility for and enrollment in specific Federal programs?
AF: Big data analytics are important because they create so much valuable insight from available data–structured and unstructured. We need analytical techniques that are sophisticated, but easy enough to use so analysts performing investigations can access and combine these different data types.
For example, the Social Security Administration is using disability claim information and looking at medical taxonomies and expected diagnoses to re-create decision-making processes to assist in identifying fraudulent claims. They could benefit from techniques and systems to transform raw, unstructured claim data into meaningful and useful information.
MeriTalk: Much of the focus has been on looking backward to identify instances that have already occurred. What types of technologies are helping agencies to identify and prevent fraud and abuse before it happens?
AF: Agencies need to act predictively rather than reactively. Many organizations are focused on determining what happened in the past and why. Predictive tools are available today that give agencies this ability.
Agencies need to combine today’s predictive analytic technologies with near-real time data ingest to determine what is happening now or is likely to happen in the near future. Tools that allow for individuals to provision new data sets without significant IT intervention and combine unstructured and traditional data are required to move agencies into being predictive, agile, and proactive. Again, the technology exists now.
MeriTalk: How is machine learning factoring into fraud, waste, and abuse identification and prevention initiatives? What’s next for machine learning in this area?
AF: Wherever the capabilities exist to integrate sensor data and Internet of Things (IoT) data for analytical work, there is an opportunity to leverage machine learning as well. Machine learning is great at sifting through enormous data sets and looking for outliers and insight we cannot get elsewhere as quickly or effectively.
For example, we need to apply machine learning to activities like modern aircraft maintenance. A fighter jet may have thousands of sensors collecting data in microsecond intervals resulting in a terabyte or two of data from just one flight. Machine algorithms can sift through huge data sets collected across an entire fleet and flag relevant outliers for a human to investigate. Applying advanced analytics to this data can identify operational trends and circumstances that can predict part and equipment failure before it happens. Engaging in this “condition-based maintenance” can prevent inefficient and wasteful use of repair and inventory resources and head off catastrophic failures before they occur.
MeriTalk: What hurdles remain in achieving real-time identification of suspect transactions or behaviors? How can agencies best address them, especially in this time of doing less with more?
AF: There are three big hurdles. First is reducing the lag between an event and detection of the event. To remove the hurdle, analysts need better, quicker access to important data.
Second is opening the world of advanced analytics to the people who are doing the investigations–fraud analysts. They need access to advanced analytical tools with pre-existing complex algorithms that enable them to plug parameters into them, rather than having to know how to write the algorithms themselves. These tools exist, but agencies have to identify them and get them into the hands of their analysts.
Third is enabling the ability to reach across various data platforms to combine data sets with greater ease. We need solutions that enable users to access and combine data regardless of where they reside and the disparate platforms involved. Again, these tools exist, but agencies need to procure and employ them.
MeriTalk: How are Teradata and its solutions helping Federal agencies attack the fraud, waste, and abuse challenge?
AF: Teradata has been helping government agency customers for more than 20 years with traditional techniques like data warehousing to combine data across multiple subject areas into a single integrated data model, enabling greater insight. We have health care solutions across the Federal and state government, such as CMS and its IDR. Several states use data warehousing tax solutions to identify tax fraud, mistakes in filings, and more. And finally, we support an enterprise data warehouse at the Postal System to help the organization run its operations more efficiently.
We also have leading-edge solutions involving advanced analytics of nontraditional data. For example, USTRANSCOM has a data warehouse that helps with materiel logistics, but also employs advanced analytics for optimizing cargo transport across available transport vehicles in the military. USTRANSCOM uses its system to marry cargo needing transport with partially loaded flights or even empty training missions to reduce the overall number of flights and optimize the efficiency of planned trips.
The Air Force and Navy use advanced Teradata analytics for pre-emptive maintenance in identifying precursor conditions of equipment failures. For example, if sensor data identifies excessive vibration in a turbine, it could indicate an imminent bearing failure. Proactive repair of the vibration or proactive replacement of affected parts helps to prevent a future broader failure.
Today and in the near future, Teradata increasingly plays a major IT and analytics consulting role, leveraging valuable intellectual property and innovative solutions from thousands of customer engagements. The technology landscape has become so complex that few organizations can operate at peak effectiveness without expert consulting guidance.